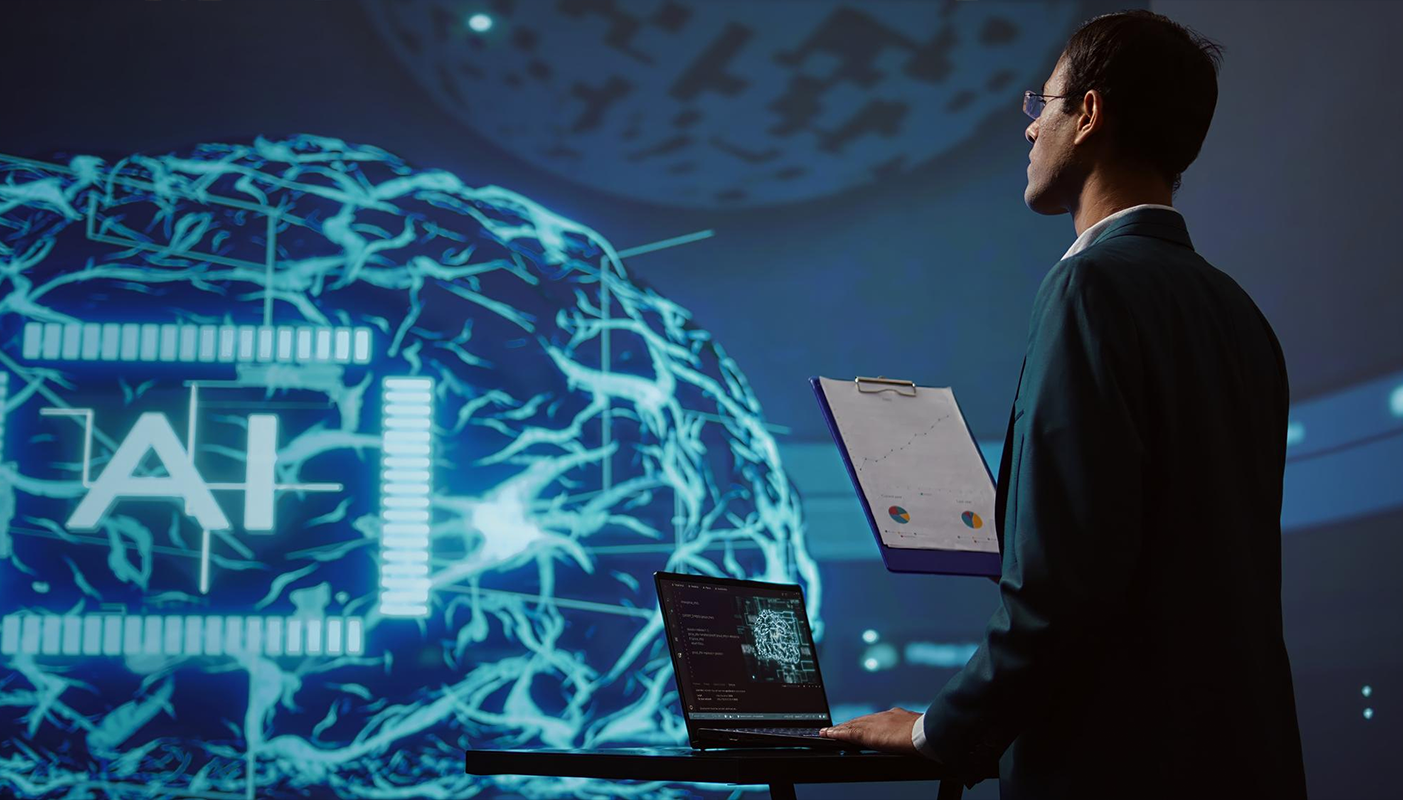
Artificial Intelligence (AI) has emerged as a transformative technology in various industries, and the life science field is no exception. AI has the potential to revolutionize the way we approach research, diagnostics, drug discovery, personalized medicine, and healthcare delivery. Artificial Intelligence encompasses the development of intelligent machines that can perform tasks requiring human-like intelligence, such as learning, reasoning, problem-solving, and decision-making. In this essay, we will explore the concept of AI, its applications in different domains, its impact on society, and the ethical considerations associated with its advancement.
Artificial Intelligence can be broadly classified into two categories: narrow AI and general AI. Narrow AI refers to systems that are designed to perform specific tasks with high proficiency, such as voice recognition, image analysis, or data analytics. General AI, on the other hand, refers to systems that possess human-like intelligence and can perform any intellectual task that a human being can. While general AI remains a long-term goal, significant advancements have been made in narrow AI applications.
APPLICATION 1.
Data Analysis and Integration: The life science field generates vast amounts of complex data from various sources, including genomic sequencing, proteomics, imaging, electronic health records, and scientific literature. AI algorithms can effectively analyze and integrate this diverse data, enabling researchers to uncover patterns, relationships, and insights that would be challenging to identify manually. AI-powered data analysis facilitates better understanding of disease mechanisms, identification of biomarkers, and optimization of treatment strategies.
APPLICATION 2.
Precision Medicine and Personalized Treatment: Precision medicine aims to provide tailored medical treatments based on an individual’s unique genetic, environmental, and lifestyle factors. AI has the potential to transform healthcare by facilitating personalized medicine. By analyzing large datasets that include genomic and clinical information, AI algorithms can identify genetic variations, biomarkers, and disease subtypes to develop tailored treatment approaches. AI can predict patient response to specific therapies, optimize drug dosages, and assist in treatment selection, leading to improved patient outcomes and reduced adverse effects.
Genomic Data Analysis: Genomic data plays a key role in precision medicine. AI algorithms can analyze genomic sequences to identify genetic variations associated with diseases, predict disease risk, and guide treatment decisions. AI helps in interpreting complex genomic data by identifying disease-associated mutations, predicting disease progression, and providing personalized treatment recommendations based on an individual’s genetic profile.
Biomarker Discovery: Biomarkers are measurable indicators of normal or abnormal biological processes, and they play a crucial role in diagnosing diseases and monitoring treatment response. AI algorithms can analyze diverse datasets, including genomic, proteomic, and clinical data, to identify novel biomarkers. This allows for early detection of diseases, accurate diagnosis, and monitoring of treatment effectiveness.
APPLICATION 3.
Predictive Modeling and Treatment Response: AI algorithms can analyze patient data, including genomic information, medical records, and clinical outcomes, to develop predictive models for treatment response. By integrating data from a large number of patients, AI can identify patterns and factors that influence treatment outcomes. This enables healthcare providers to predict how an individual will respond to a particular treatment and tailor interventions accordingly.
APPLICATION 4.
Drug Discovery and Development: Drug discovery and development is a complex and time-consuming process that involves identifying potential drug candidates, optimizing their properties, and testing their safety and efficacy. Artificial Intelligence has emerged as a powerful tool in this field, revolutionizing the way new drugs are discovered and developed. AI has transformed the field of drug discovery and development by accelerating the process, optimizing drug design, and improving treatment outcomes. The ability of AI to analyze vast amounts of data, predict drug-target interactions, optimize clinical trials, and repurpose existing drugs has the potential to revolutionize the pharmaceutical industry. However, challenges such as data quality, interpretability, and regulatory considerations must be addressed to fully harness the power of AI in drug discovery and development.
Virtual Screening and Target Identification: AI algorithms can perform virtual screening of large libraries of compounds to identify potential drug candidates. By analyzing molecular structures, properties, and interactions, AI can predict the likelihood of a compound binding to a specific target, such as a protein or enzyme associated with a disease. This helps in identifying promising drug candidates for further investigation.
Drug Design and Optimization: AI plays a crucial role in designing and optimizing drug candidates. Machine learning algorithms can analyze large datasets of chemical structures, biological activities, and pharmacological properties to predict the optimal properties of a drug, such as potency, selectivity, and toxicity. This enables researchers to optimize lead compounds and design novel molecules with desired characteristics.
Prediction of Drug-Target Interactions: AI algorithms can predict the interactions between drugs and their target molecules, providing insights into the mechanism of action and potential side effects. By analyzing large databases of drug-target interactions, AI can identify new drugtarget associations and repurpose existing drugs for different indications. This approach accelerates the drug discovery process and expands the possibilities for drug development.
Toxicity Prediction: AI algorithms can predict the potential toxicity of drug candidates, minimizing the risk of adverse effects during clinical trials. By analyzing chemical structures, biological data, and known toxicity profiles, AI models can identify potential toxicities, such as liver or cardiac toxicity, before a drug candidate enters clinical trials. This helps in prioritizing safe and effective drug candidates and reducing the attrition rate during development.
Clinical Trial Optimization: AI can optimize the design and execution of clinical trials, making them more efficient and cost-effective. AI algorithms can analyze patient data, genomic profiles, and treatment outcomes to identify subpopulations that are more likely to respond positively to a drug. This enables the selection of suitable patient cohorts for clinical trials, leading to more accurate results and faster evaluation of drug efficacy.
Real-time Data Analysis and Decision Support: AI algorithms can analyze real-time patient data from electronic health records, wearable devices, and other sources to support clinical decision-making. By analyzing patient characteristics, treatment histories, and clinical outcomes, AI can provide personalized treatment recommendations, assist in adverse event detection, and optimize dosing regimens. This enhances patient care and improves treatment outcomes.
Repurposing Existing Drugs: AI algorithms can identify new therapeutic uses for existing drugs by analyzing large datasets of drug properties, disease associations, and biological pathways. This approach, known as drug repurposing or repositioning, can significantly shorten the development timeline and reduce costs, as the safety profiles of repurposed drugs are already known.
APPLICATION 5.
Imaging and Diagnostics: Artificial Intelligence has revolutionized the field of medical imaging and diagnostics. By leveraging advanced algorithms and machine learning techniques, AI has the potential to enhance the accuracy, efficiency, and accessibility of diagnostic imaging. AI algorithms can analyze medical images, such as X-rays, MRIs, and CT scans, with remarkable accuracy and speed. AI-based image analysis can aid in early detection, diagnosis, and characterization of diseases. For example, AI can detect cancerous lesions, identify patterns of disease progression, and assist radiologists in making more accurate diagnoses. AI algorithms can also integrate imaging data with clinical and genomic information to provide a comprehensive assessment of a patient’s health status.
Image Analysis and Interpretation: AI algorithms excel in analyzing and interpreting medical images, such as X-rays, CT scans, MRI scans, and mammograms. These algorithms can detect abnormalities, segment anatomical structures, and assist in the diagnosis of various conditions. By leveraging deep learning techniques, AI can learn from vast datasets and provide accurate and consistent interpretations, aiding radiologists in their decision-making process.
Early Detection and Diagnosis: AI has the potential to improve early detection and diagnosis of diseases. By analyzing medical images, AI algorithms can detect subtle abnormalities or patterns that may be missed by human observers. This can lead to earlier detection of diseases, such as cancer, and enable prompt initiation of treatment, potentially improving patient outcomes.
Radiomics and Quantitative Analysis: AI algorithms can extract quantitative features from medical images, leading to the field of radiomics. Radiomics involves the analysis of these features to identify imaging biomarkers that correlate with clinical outcomes. This approach allows for a more comprehensive and objective assessment of diseases, aiding in diagnosis, treatment planning, and monitoring of treatment response.
Decision Support Systems: AI-powered decision support systems can assist radiologists in their interpretation and diagnosis. These systems can analyze patient data, including medical images, electronic health records, and clinical data, to provide additional insights and recommendations. AI algorithms can flag suspicious findings, suggest differential diagnoses, and provide evidence-based guidelines, aiding radiologists in making more accurate and informed decisions.
Workflow Optimization: AI can optimize the workflow in imaging departments and clinics. By automating routine tasks, such as image pre-processing and triaging, AI algorithms can free up radiologists’ time and enable them to focus on more complex cases. AI can also prioritize urgent cases, reducing waiting times and improving patient care.
Telemedicine and Remote Imaging: AI has the potential to facilitate telemedicine and remote imaging, especially in underserved areas or during emergencies. AI algorithms can analyze medical images in real-time, enabling remote specialists to provide timely and accurate diagnoses. This can improve access to specialized care, reduce the need for patient travel, and enhance healthcare delivery in remote or resource-limited settings.
APPLICATION 6.
Robotics and Surgical Assistance: AI is revolutionizing surgical assistance by improving preoperative planning, enhancing visualization, enabling robotic-assisted surgery, providing decision support, and facilitating surgical training and skill assessment. The integration of AI into surgical practice holds great potential for improving surgical precision, patient outcomes, and overall healthcare delivery. However, addressing challenges related to data quality, ethical considerations, and training is crucial to harness the full benefits of AI in surgical assistance. With continued advancements and collaboration between surgeons and AI experts, the future of surgical assistance looks promising and transformative.
Preoperative Planning: AI algorithms can analyze patient data, including medical images, electronic health records, and genomic profiles, to assist in preoperative planning. By integrating and interpreting these data, AI can provide surgeons with insights into the patient’s anatomy, pathology, and potential challenges. This enables surgeons to develop personalized surgical strategies, optimize incision and approach, and improve surgical outcomes.
Image Analysis and Augmented Visualization: AI algorithms can analyze medical images, such as CT scans and MRI scans, to provide detailed insights and enhance visualization during surgery. AI-powered image analysis can segment anatomical structures, identify important landmarks, and highlight areas of interest. This augmented visualization helps surgeons navigate complex anatomical structures and perform procedures with greater precision, reducing the risk of complications.
Robotic Surgery: AI plays a crucial role in robotic-assisted surgery, where robots controlled by surgeons perform procedures with enhanced precision and dexterity. AI algorithms enable real-time feedback and automation of certain tasks, such as suturing or tissue manipulation. Robotic-assisted surgery offers several advantages, including smaller incisions, reduced blood loss, faster recovery, and improved cosmetic outcomes. AI ensures that the robots operate with high accuracy, enabling surgeons to perform complex procedures with improved outcomes.
Intraoperative Decision Support: During surgery, AI can provide real-time decision support to assist surgeons in making critical decisions. By analyzing live data from surgical instruments, patient vitals, and imaging systems, AI algorithms can provide insights and recommendations. For example, AI can detect abnormal tissue characteristics, warn of potential complications, or suggest alternative approaches. This assists surgeons in adapting their techniques, reducing errors, and optimizing patient safety.
Predictive Analytics and Outcome Assessment: AI algorithms can analyze large datasets of patient records and surgical outcomes to develop predictive models for surgical procedures. By identifying patterns and factors that influence outcomes, AI can provide insights into surgical risks, postoperative complications, and patient recovery. This information can help surgeons in patient selection, personalized treatment planning, and informed consent discussions with patients.
Surgical Training and Skill Assessment: AI-based surgical assistance systems can play a vital role in surgical training and skill assessment. By analyzing surgical videos and data, AI algorithms can provide objective assessments of surgical performance, highlighting areas for improvement and guiding skill development. AI can also create virtual simulations for surgeons to practice and refine their techniques in a risk-free environment.
APPLICATION 7.
Clinical Decision Support Systems: AI-powered clinical decision support systems assist healthcare professionals in making accurate and evidence-based decisions. These systems can analyze patient data, medical records, and scientific literature to provide real-time recommendations on diagnostics, treatment options, and personalized care plans. AI algorithms can help detect drug interactions, predict disease progression, and even identify potential adverse events, enabling proactive intervention and improved patient safety.
APPLICATION 8.
Genomics and Precision Genomic Medicine: Genomic data is incredibly complex and challenging to interpret. AI plays a vital role in genomics research and precision genomic medicine. AI algorithms can analyze vast genomic datasets to identify disease-associated genetic variations, predict disease risk, and guide treatment decisions based on an individual’s genetic profile. AI can also assist in interpreting the functional significance of genetic variants, improving our understanding of genetic diseases, and facilitating targeted therapies.
APPLICATION 9.
Regulatory Compliance and Drug Safety: AI technologies can aid in ensuring regulatory compliance and drug safety. AI algorithms can analyze vast amounts of preclinical and clinical data to detect safety signals, adverse effects, and potential drug-drug interactions. These algorithms can assist in identifying drug candidates with the highest safety profiles and guide regulatory decision-making.
ETHICAL CONSIDERATIONS OF AI IN LIFE SCIENCE RESEARCH FIELD
AI holds tremendous potential in the life science research field, but it is essential to address the ethical considerations associated with its use. Privacy protection, mitigating biases, ensuring transparency and accountability, obtaining informed consent, and addressing social and economic implications are all crucial ethical considerations. By addressing these concerns and integrating ethical principles into AI development and implementation, we can harness the full potential of AI in lifescience research while upholding patient rights, fairness, and societal wellbeing.
1. Data Privacy and Informed Consent: AI in life science research relies heavily on accessing and analyzing vast amounts of personal health data. Protecting the privacy and confidentiality of individuals’ health information is paramount. Researchers must adhere to strict data protection regulations and obtain informed consent from participants. Data anonymization and de-identification techniques should be implemented to minimize the risk of re-identification and unauthorized access to sensitive information.
2. Bias and Fairness: AI algorithms are trained on large datasets, and if those datasets are biased, it can lead to biased outcomes and perpetuate discrimination. Care must be taken to ensure that training datasets are representative and diverse, avoiding biases related to race, gender, or other protected characteristics. Regular audits and evaluations of AI systems should be conducted to detect and mitigate any biases that may arise.
3. Transparency and Explainability: AI algorithms often operate as black boxes, making it challenging to understand the underlying decision-making process. It is important to strive for transparency and develop AI systems that are explainable. Researchers should work towards building models that provide clear explanations of their outputs, enabling researchers, healthcare professionals, and patients to understand how decisions are made and ensuring accountability.
4. Accountability and Responsibility: AI in life science research raises questions about accountability and responsibility. Researchers and developers should be accountable for the outcomes and consequences of their AI systems. Establishing clear lines of responsibility, developing ethical guidelines, and ensuring proper training and oversight are essential to maintain accountability and uphold ethical standards in the field.
5. Human Subjects and Informed Consent: When AI systems are used in research involving human subjects, informed consent becomes a critical ethical consideration. Participants must be fully informed about the use of AI, its potential risks and benefits, and the implications for their privacy. Researchers should ensure that participants have the right to withdraw their consent at any time and that their data is handled with the utmost care and protection.
6. Impact on Employment and Healthcare Professionals: The integration of AI in life science research has the potential to impact employment and the roles of healthcare professionals. It is essential to consider the potential effects on jobs and the need for retraining or upskilling healthcare professionals. Ensuring a smooth transition and providing support to those affected will be crucial to address any ethical concerns arising from the introduction of AI in the workforce.
7. Intellectual Property and Open Science: AI-driven research generates vast amounts of data and knowledge. Ethical considerations include balancing the need for intellectual property protection with the importance of open science and knowledge sharing. Researchers should strive to find a balance that promotes collaboration, transparency, and the advancement of scientific knowledge while respecting the intellectual property rights of individuals and organizations.
FUTURE PROSPECTS
The future of AI in the life science field holds immense promise. Advancements in AI technology, such as explainable AI and reinforcement learning, will further enhance the interpretability, transparency, and reliability of AI models. Integration of AI with emerging technologies like blockchain and Internet of Things (IoT) will enable secure and seamless data sharing, improving collaboration and accelerating research and development. Furthermore, AI can be applied to the field of synthetic biology, where it can aid in the design and engineering of novel biomolecules and synthetic organisms.
CONCLUSION
Artificial Intelligence has the potential to revolutionize the life science field by transforming research, diagnostics, drug discovery, and healthcare delivery. AI-powered data analysis, precision medicine, drug discovery, imaging analysis, clinical decision support systems, genomics, and regulatory compliance are just a few areas where AI is making a significant impact. As AI technology continues to advance, it will play an increasingly important role in improving patient outcomes, enabling personalized treatments, and driving scientific discoveries. The integration of AI with other emerging technologies holds promise for further innovation and transformative advancements in the life science field
Biotechnologist, VETA Genomics